Achieving Product-Market Fit in Voice AI

Before we dive in, here are some examples for context. Check out this CNN reporter astounded by Vapi and Groq’s AI chip here. Check out one of our demos showing Lightspeed Math-bot solving arithmetic problems with impressive latency here (note: LLM’s typically have issues with these types of queries). And check out the conversational intelligence in this demo featuring Winston Churchill-bot here.
A Primer on PMF
There are various frameworks through which to assess product-market fit. Since I am a lean product aficionado, we’ll approach this as outlined in The Lean Product Playbook. First, we need a basic definition of the space. Per ChatGPT 4:
Voice AI, or Voice-Activated Artificial Intelligence, refers to advanced AI systems capable of understanding, processing, and responding to human speech. This technology combines several fields of AI, such as natural language processing (NLP), machine learning (ML), and speech recognition, to enable machines to interact with humans in a way that mimics natural conversation.
The graphic above shows the Product Market-Fit Pyramid which forms the basis of lean product theory. Steps two and three are the ones we want to focus on: Identify Underserved Customer Needs and Define Your Value Proposition. It is where these two intersect that a product achieves PMF.
Once the target customers have been identified and a hypothesis formed around how a product could meet their needs, we move on to the specific underserved needs corresponding to a good market opportunity. This is where we divide the hypothesis into the “problem space” and the “solution space”.
The problem space represents everything related to the customer problems and needs that a product will be meeting. The solution space encompasses everything that is done to create a product that will meet those needs: building, developing, engineering, etc.
Finally, we have to define the value proposition. This entails answering two questions: what benefits is the product/product features providing, and how is the product superior to competitors’ solutions?
Breaking it all down: PMF is defined as how well the underserved needs in the problem space are met by the product features in the solution space; both in terms of benefits provided to the customer, and whether the product provides these benefits in a way that outperforms the competition.
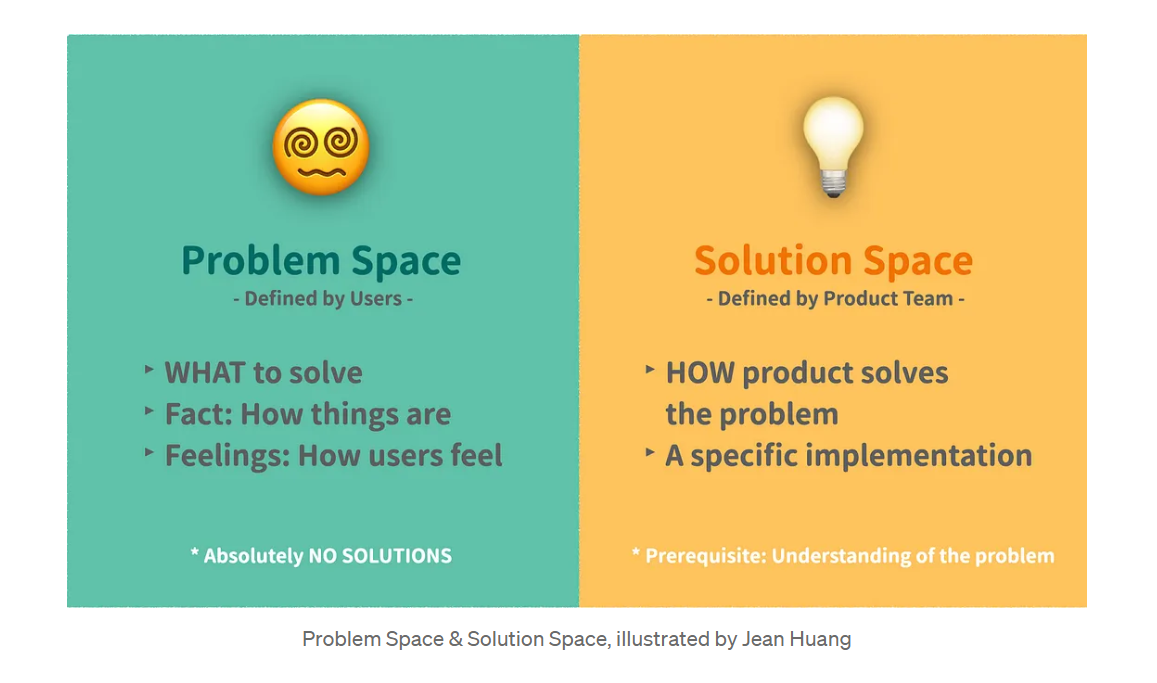
PMF and Voice AI
Problem Space
- Need conversational intelligence, e.g. interruptions
- Need low latency to properly simulate human conversation
- Need processing speed enough to prevent gaps and periods of silence
Solution Space
- ML support to learn and improve models from usage data
- Infrastructure and pipeline optimization to ensure low enough latency
- AI chip sufficiently fast to enable conversational flow
As we can see, achieving PMF here is about much more than just having an operational voice assistant. See this previous blog post to learn more about the implications of conversational intelligence and latency for voice AI.
Tying It All Together with Vapi
Looking at the problem space and solution space above, it’s clear that Vapi has a strong PMF with our voice AI platform. We continue to push ahead in tweaking our models using various ML approaches (we'll delve into this in a later post). And thanks to the Groq AI chip, we’re working at unprecedented speeds. Finally, we’re constantly optimizing our pipeline for lower latency.
But don’t take my word for it. Presumably, if you're interested in a voice AI solution, factors like latency and performance are critical. Come visit our dashboard and assess for yourself whether or not we're meeting these needs for customers.